The Impact of AI on Renewable Energy Management
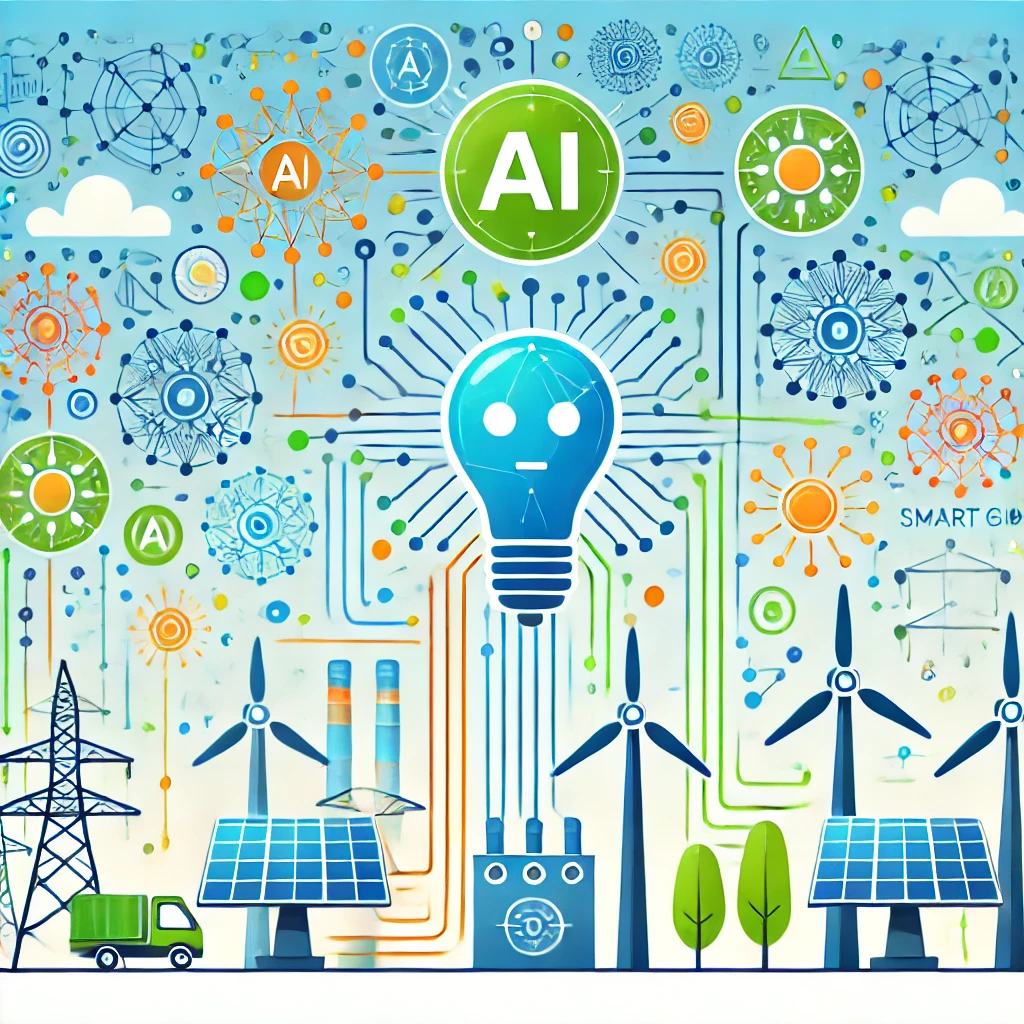
Artificial intelligence (AI) is playing a transformative role in the management and optimization of renewable energy. With growing concerns about climate change and the need for sustainable energy solutions, AI offers new ways to enhance the efficiency, integration, and reliability of renewable energy sources. From predicting energy demand to optimizing grid performance, AI-driven solutions are reshaping the energy landscape and making renewable energy management smarter and more effective.
1. Predictive Analytics for Energy Forecasting
AI algorithms excel at analyzing large datasets and identifying patterns, making them ideal for predicting energy generation and consumption. In renewable energy management, AI can forecast the output of renewable sources like solar, wind, and hydroelectric power by considering factors such as weather conditions, historical data, and geographical location.
Examples of predictive analytics in action:
- Wind power forecasting: AI models can predict wind patterns, helping wind farms optimize turbine performance and energy output. By accurately forecasting wind speeds and directions, energy producers can better plan when to ramp up or scale down operations.
- Solar energy forecasting: AI can analyze cloud cover, temperature, and sunlight intensity to estimate solar panel output, ensuring that energy supply aligns with demand and minimizing waste.
By using AI for predictive analytics, energy producers can improve the reliability of renewable energy sources and make better-informed decisions about energy distribution.
2. Enhancing Grid Stability and Integration
One of the challenges with renewable energy is its intermittent nature; solar and wind power depend on weather conditions, which can be unpredictable. AI helps to address these challenges by optimizing the integration of renewable energy sources into the power grid, ensuring grid stability and minimizing disruptions.
Ways AI enhances grid stability:
- Load balancing: AI algorithms can analyze real-time data to distribute electricity more efficiently across the grid. This includes managing peak loads and distributing excess renewable energy to storage systems or other regions where it is needed.
- Demand response management: AI can predict fluctuations in energy demand and adjust the output of renewable sources accordingly. This helps to prevent blackouts and ensures a continuous energy supply, even during peak demand periods.
By enhancing grid stability, AI enables a smoother transition to renewable energy and helps reduce reliance on traditional fossil fuel-based power plants.
3. Optimizing Energy Storage Systems
Energy storage is essential for balancing supply and demand in renewable energy systems. AI plays a crucial role in optimizing energy storage technologies, such as batteries, by predicting energy demand, managing charging cycles, and preventing overuse.
Examples of AI-driven energy storage optimization:
- Battery management: AI can monitor the health and performance of batteries in real time, extending their lifespan by optimizing charging and discharging cycles. This ensures that stored energy is used efficiently and minimizes the need for frequent replacements.
- Energy arbitrage: AI algorithms can identify the best times to store and release energy based on electricity prices, grid demand, and renewable energy output. This allows energy producers to maximize revenue by selling stored energy when demand (and prices) are high.
By improving the efficiency of energy storage systems, AI contributes to more reliable renewable energy management and reduces the overall costs of energy production.
4. Smart Maintenance and Asset Management
Renewable energy assets, such as wind turbines and solar panels, require regular maintenance to ensure optimal performance. AI-driven predictive maintenance systems can identify potential issues before they become critical, allowing for proactive repairs and reducing downtime.
AI applications in maintenance:
- Fault detection: AI can analyze data from sensors installed on wind turbines and solar panels to detect anomalies and predict equipment failures. This enables maintenance teams to address issues early, preventing costly repairs and energy loss.
- Resource allocation: AI can optimize the deployment of maintenance crews by predicting which assets are most likely to require attention. This minimizes travel time, reduces maintenance costs, and ensures that critical infrastructure remains operational.
Through smart maintenance, AI helps to increase the reliability and lifespan of renewable energy assets, ultimately leading to more efficient energy production.
5. Supporting Decentralized Energy Systems
The rise of decentralized energy systems, such as microgrids and distributed energy resources (DERs), is changing the way electricity is generated and consumed. AI facilitates the management of decentralized energy systems by optimizing local energy production, storage, and consumption.
Examples of AI in decentralized energy management:
- Microgrid optimization: AI can coordinate the operation of multiple energy sources within a microgrid, including solar panels, wind turbines, and batteries. This allows for efficient energy sharing between households or communities, reducing dependency on centralized power grids.
- Peer-to-peer energy trading: AI algorithms can enable peer-to-peer energy trading, where households with excess solar power can sell energy to their neighbors. By matching buyers and sellers in real time, AI helps to create a more flexible and resilient energy market.
AI’s role in decentralized energy management supports the development of sustainable, community-driven energy solutions that are better equipped to handle local needs.
Challenges and Considerations
Despite its potential, integrating AI into renewable energy management presents some challenges:
- Data Privacy and Security: AI systems require large amounts of data to function effectively, raising concerns about data privacy and cybersecurity. Ensuring that data is protected and used responsibly is crucial.
- High Implementation Costs: AI deployment in energy management can be costly, especially for small-scale operators. The cost of acquiring, training, and maintaining AI systems can be a barrier to entry.
- Algorithm Bias and Accuracy: AI models must be trained on diverse datasets to avoid bias and ensure accuracy. Inaccurate predictions or biased algorithms could lead to suboptimal energy management decisions.
Conclusion
AI is playing a crucial role in the management of renewable energy, from predictive analytics and grid stability to energy storage optimization and smart maintenance. By addressing the challenges associated with renewable energy’s intermittent nature, AI is helping to make sustainable energy solutions more reliable and efficient. As the technology continues to evolve, AI-driven innovations will further support the transition to a cleaner, smarter, and more resilient energy future.