Understanding the Ethics of AI Development
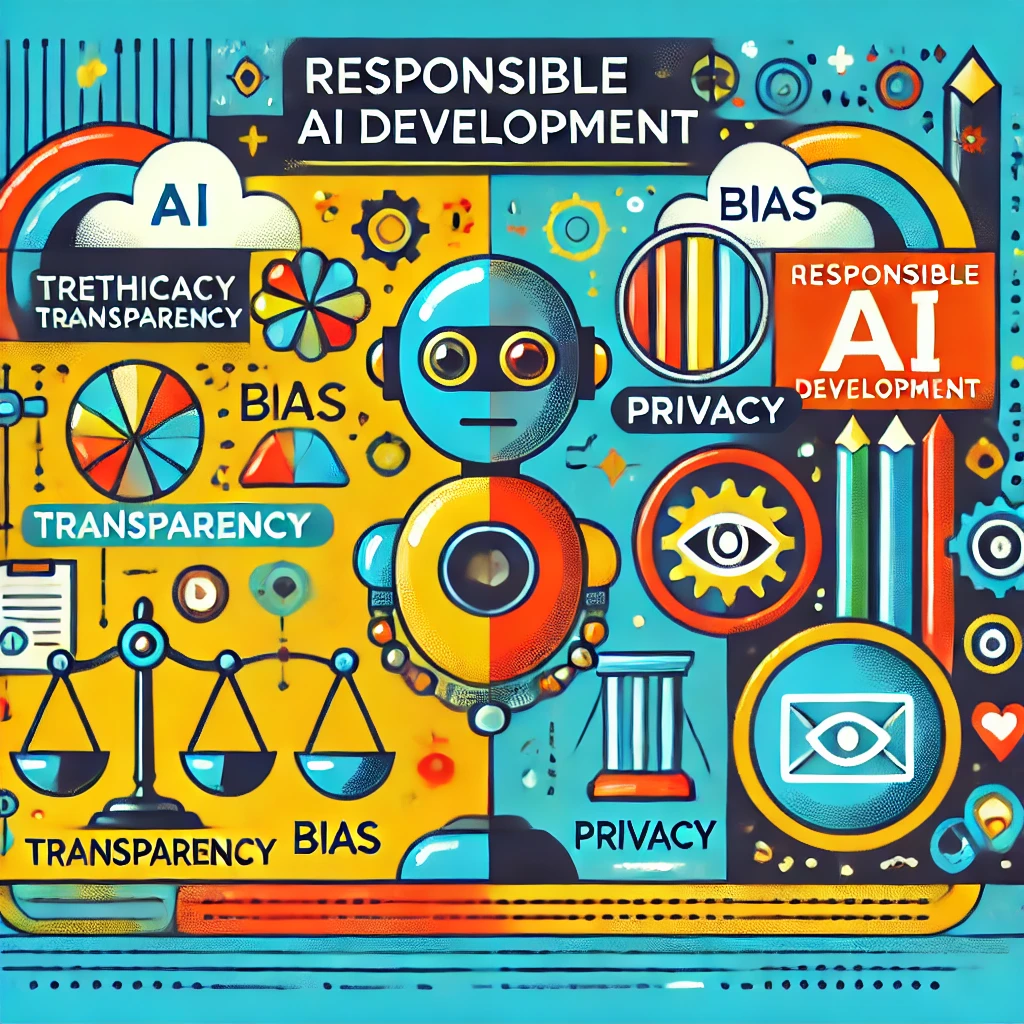
As artificial intelligence (AI) continues to advance, it brings numerous benefits, such as improved efficiency, automation, and data-driven decision-making. However, with these advantages come ethical challenges that need to be addressed to ensure responsible AI development. The ethical considerations surrounding AI encompass issues like privacy, bias, accountability, and transparency. Understanding these concerns is essential for building AI systems that are not only effective but also aligned with societal values and principles.
1. Privacy Concerns
AI systems often rely on vast amounts of data for training and decision-making, including personal information collected from users. While this data enables AI to improve its performance and provide personalized experiences, it also raises significant privacy concerns. The collection, storage, and use of personal data can lead to the risk of data breaches, unauthorized access, and misuse of sensitive information.
Examples of privacy issues:
- Facial recognition technologies can track individuals without their consent, raising questions about surveillance and personal freedom.
- AI-powered recommendation systems use personal data to predict user preferences, but this can result in intrusive targeted advertising.
To address privacy concerns, developers need to implement data protection measures such as anonymization, data minimization, and encryption. Adhering to privacy regulations like the General Data Protection Regulation (GDPR) is also essential to safeguard user data.
2. Algorithmic Bias
AI algorithms can reflect and even amplify existing biases present in the data used to train them. This can lead to unfair and discriminatory outcomes, particularly in areas like hiring, law enforcement, and healthcare, where biased AI systems can disproportionately affect marginalized groups. Bias in AI arises from various sources, such as biased training data, biased algorithmic design, or human prejudice in data labeling.
Examples of algorithmic bias:
- AI-driven hiring tools may favor certain demographics over others if the training data reflects historical hiring trends that exhibit bias.
- Predictive policing algorithms may target specific communities based on biased crime data, perpetuating discriminatory practices.
Mitigating algorithmic bias requires developers to use diverse and representative datasets, regularly audit AI systems for bias, and involve multidisciplinary teams in the development process to identify potential ethical pitfalls.
3. Accountability and Responsibility
When AI systems make decisions that affect people’s lives, determining who is accountable for those decisions can be challenging. If an AI-driven system makes an incorrect or harmful decision, is the developer, the company deploying the AI, or the algorithm itself responsible? This issue of accountability is particularly concerning in areas like autonomous vehicles, healthcare, and financial services.
Accountability considerations:
- Autonomous vehicles may be involved in accidents where it is unclear whether the responsibility lies with the car manufacturer, the software developer, or the user.
- AI systems used in healthcare to diagnose medical conditions could result in liability issues if the diagnosis is incorrect or leads to harm.
Establishing clear frameworks for accountability is crucial. It may involve creating regulatory standards for AI deployment, requiring human oversight in critical decisions, and implementing mechanisms for recourse when AI systems cause harm.
4. Transparency and Explainability
AI systems, especially those based on complex machine learning models, are often referred to as “black boxes” because their decision-making processes are not easily interpretable. The lack of transparency can make it difficult to understand how an AI system arrived at a particular decision, which raises ethical concerns when AI is used in sensitive areas such as criminal justice, healthcare, and finance.
Challenges with transparency:
- Black-box algorithms used in credit scoring may deny loans to individuals without providing a clear explanation for the decision.
- AI-driven sentencing tools in the criminal justice system may recommend harsher penalties based on factors that are not transparent or justifiable.
To address these concerns, AI developers can focus on creating explainable AI (XAI) systems that provide interpretable results. Techniques like model-agnostic interpretability and feature importance analysis can help users understand AI decision-making processes. Regulatory bodies may also mandate the use of explainable algorithms in high-stakes applications.
5. The Impact on Employment
AI and automation are reshaping the job market by automating repetitive tasks and enabling new types of work. While this can increase efficiency, it can also lead to job displacement and economic inequality. Some jobs may become obsolete, requiring workers to adapt to new roles or upskill to remain employable.
Examples of AI’s impact on employment:
- Automated customer service chatbots reduce the need for human agents, potentially leading to job losses in call centers.
- AI-powered tools in manufacturing may replace manual labor for tasks like quality inspection and assembly.
To mitigate the negative impact on employment, governments, and organizations can invest in retraining programs, promote lifelong learning, and create policies that support workers affected by AI-driven automation. Ensuring a fair transition to an AI-powered economy is crucial for social stability and inclusivity.
6. Autonomous Decision-Making and Ethical Dilemmas
AI systems capable of making autonomous decisions raise ethical questions, particularly when those decisions involve life-and-death situations. For example, autonomous vehicles may face scenarios where they need to make split-second decisions that could harm passengers or pedestrians. These ethical dilemmas, often referred to as the “trolley problem” in AI, require careful consideration of moral principles.
Ethical dilemmas in autonomous systems:
- Should an autonomous vehicle prioritize the safety of its passengers or pedestrians in a potential collision?
- How should healthcare AI systems balance the trade-offs between potential treatment outcomes and patient quality of life?
Addressing these dilemmas requires the involvement of ethicists, policymakers, and the public to establish guidelines for how AI systems should behave in morally complex situations.
Conclusion
The ethical considerations in AI development are critical to ensuring that the technology benefits society while minimizing harm. Addressing issues such as privacy, bias, accountability, transparency, employment, and autonomous decision-making requires a collaborative approach involving developers, regulators, and society at large. By implementing responsible AI practices, such as data protection, bias mitigation, and transparency, developers can create ethical AI systems that align with societal values and contribute to a fairer, safer, and more inclusive future.