The Role of AI in Fraud Detection
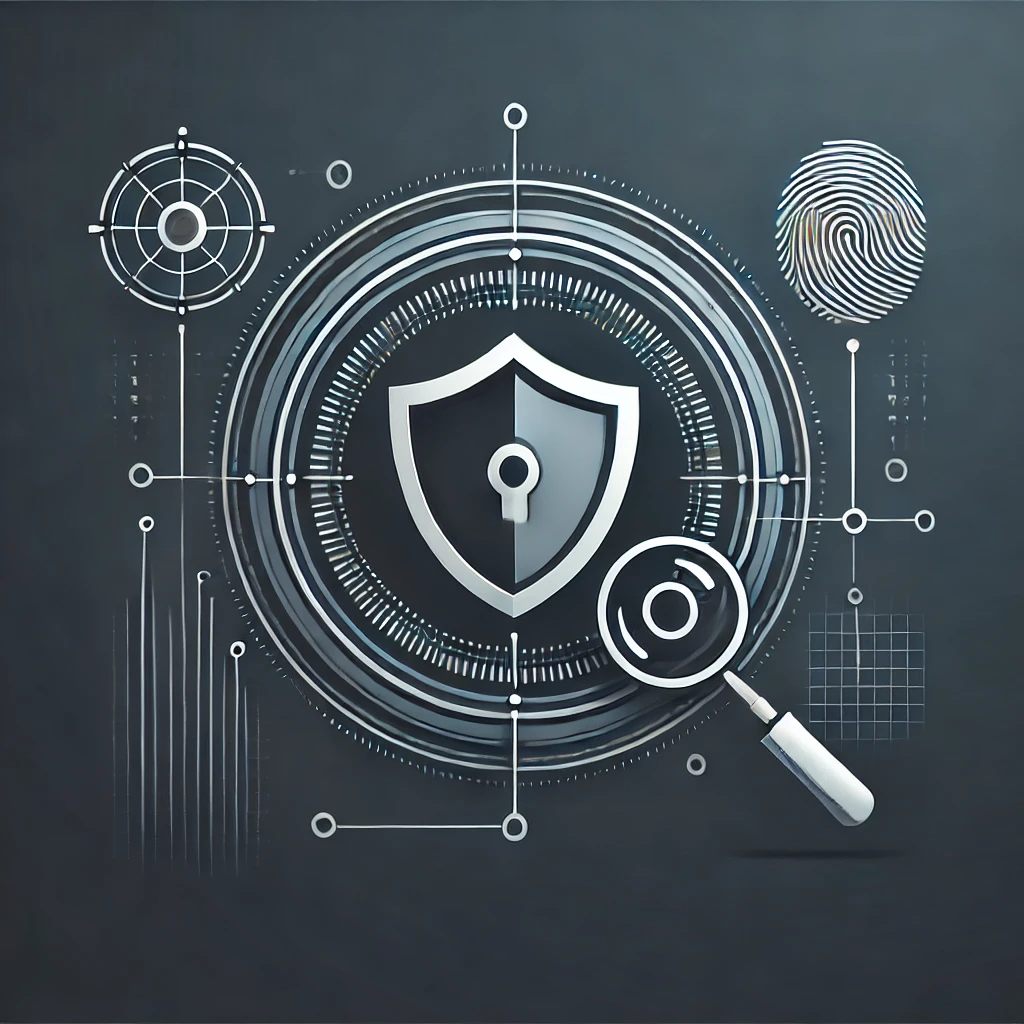
Fraud detection has become one of the most pressing concerns for businesses across various industries, especially in financial services, e-commerce, healthcare, and insurance. The rapid digital transformation of businesses has brought about new opportunities but also a surge in fraudulent activities. Traditional fraud detection systems, based on predefined rules and patterns, are often insufficient in identifying sophisticated, evolving, and subtle fraud schemes. This is where Artificial Intelligence (AI) comes into play, revolutionizing the way organizations detect and combat fraud. AI, particularly machine learning (ML) and deep learning (DL), offers powerful tools for detecting fraudulent activities in real-time, minimizing losses, and enhancing overall security.
In this article, we will explore the role of AI in fraud detection, how AI-driven systems work, the types of fraud they can help prevent, and the benefits of using AI for fraud prevention.
1. How AI Enhances Fraud Detection
Fraud detection traditionally relied on rule-based systems where fraud patterns were manually defined by experts. These systems could not adapt quickly to emerging fraud tactics, making them vulnerable to new types of fraudulent activities. AI, particularly machine learning (ML), transforms this process by allowing systems to automatically learn from data and continuously improve detection capabilities.
AI models can analyze vast amounts of transactional data, identify complex patterns, and detect anomalies without human intervention. By continuously learning from data, AI-powered systems can quickly adapt to new fraud tactics and detect even the most sophisticated fraud attempts. This is especially important in fast-paced environments where fraud can evolve rapidly, such as online transactions or digital banking.
2. Machine Learning for Fraud Detection
At the heart of AI-driven fraud detection is machine learning, a subset of AI that enables systems to learn from historical data and improve their performance over time. ML algorithms can be trained using large datasets of both legitimate and fraudulent transactions, helping the system to recognize patterns that distinguish between normal behavior and fraudulent activity.
There are two main types of machine learning techniques used in fraud detection:
- Supervised Learning: In supervised learning, the model is trained using labeled data that includes both fraudulent and non-fraudulent examples. The algorithm learns to classify future transactions based on these patterns. For example, in credit card fraud detection, a supervised learning model would be trained on a dataset containing both legitimate and fraudulent transactions, allowing it to recognize patterns in spending behavior that typically indicate fraud.
- Unsupervised Learning: Unsupervised learning, on the other hand, is used when labeled data is not available. In this case, the model analyzes data to identify unusual patterns or outliers that deviate from normal behavior. This approach is particularly useful in detecting new types of fraud that have not been encountered before, as the system does not rely on predefined labels.
Machine learning models can be further enhanced with techniques like ensemble learning, which combines multiple models to improve accuracy and reduce errors, and deep learning, which utilizes neural networks to detect complex, non-linear patterns in large datasets.
3. Types of Fraud AI Helps Detect
AI has proven to be a powerful tool in identifying a wide range of fraudulent activities across various industries. Some of the most common types of fraud that AI can help detect include:
- Credit Card Fraud: AI systems can detect abnormal spending patterns, such as unusual geographic locations or sudden spikes in transaction amounts. Machine learning models can also identify when a card is being used by someone other than the owner by analyzing patterns like the frequency of transactions or the type of purchases made.
- Insurance Fraud: In the insurance industry, fraud can take many forms, such as exaggerated claims or the submission of false information. AI can analyze claims data to identify inconsistencies or suspicious behavior, such as frequent claims from the same individual or unusually high claim amounts. It can also detect fake documentation, such as doctored medical records or vehicle damage reports.
- E-commerce Fraud: Online retailers are frequent targets for fraud, including payment fraud, account takeover, and identity theft. AI systems can flag suspicious activities such as multiple orders from the same account in a short time, abnormal IP addresses, or irregular shipping addresses. Machine learning can also detect bot attacks or fake reviews that are often used to manipulate the purchasing process.
- Banking Fraud: AI is crucial in monitoring banking transactions for fraud, such as money laundering or account takeover. AI models can track unusual transaction behavior, such as large withdrawals or transfers that do not align with typical customer patterns. AI-driven systems can also analyze text-based data, such as emails or phone calls, to identify signs of phishing attempts or social engineering attacks.
- Healthcare Fraud: Healthcare providers and insurers face significant risks from fraud, such as billing for services not rendered or falsifying patient information. AI can analyze billing patterns, identify duplicate claims, and detect anomalies in patient treatment records. Machine learning models can also flag suspicious healthcare providers who submit claims at an unusually high rate or for services that are inconsistent with their specialties.
4. Benefits of AI in Fraud Detection
The implementation of AI in fraud detection offers several key benefits:
- Real-time Detection: One of the primary advantages of AI in fraud detection is its ability to analyze transactions in real-time. Traditional systems often have delays in detecting fraud, allowing fraudulent activities to escalate before being caught. AI models, on the other hand, can instantly identify suspicious transactions and trigger alerts, preventing or minimizing potential losses.
- Adaptability: AI systems continuously learn from new data, enabling them to adapt to evolving fraud tactics. As fraudsters find new ways to exploit vulnerabilities, AI models can detect these new schemes by identifying patterns that may have gone unnoticed by traditional systems. This ability to adapt makes AI a highly effective tool in the fight against emerging fraud.
- Accuracy and Efficiency: AI-driven fraud detection systems can analyze vast amounts of data far more efficiently than human analysts. By automating the detection process, AI reduces the number of false positives (i.e., legitimate transactions flagged as fraud) and false negatives (i.e., fraudulent transactions missed by the system), improving the accuracy and efficiency of fraud detection.
- Cost-Effectiveness: Although implementing AI-driven fraud detection systems requires an initial investment in technology, it can ultimately save organizations money by preventing fraud before it occurs. AI can reduce the need for manual monitoring, allowing businesses to allocate resources more effectively and focus on higher-level strategic activities.
- Enhanced Customer Experience: In addition to improving security, AI can enhance the customer experience by reducing friction in the fraud detection process. For example, AI-powered systems can flag suspicious transactions and verify their legitimacy with minimal disruption to the customer. This proactive approach to fraud detection ensures that customers are not inconvenienced by fraudulent activity while maintaining a seamless experience.
5. Challenges and Considerations in Implementing AI for Fraud Detection
Despite its many benefits, implementing AI in fraud detection comes with challenges. These include:
- Data Quality and Privacy Concerns: AI models rely on high-quality data to make accurate predictions. Ensuring that the data used for training is clean, accurate, and unbiased is essential. Moreover, privacy concerns must be addressed, especially when sensitive data such as financial or medical records are involved. Businesses must adhere to regulations like GDPR and HIPAA to ensure that customer data is protected.
- Integration with Existing Systems: AI-powered fraud detection systems need to be integrated with existing infrastructure, which can be complex and costly. Organizations must ensure that AI solutions work seamlessly with other tools, such as transaction processing systems and customer databases, to create a cohesive fraud prevention strategy.
- Model Transparency: While AI can provide powerful insights into fraud patterns, the “black-box” nature of some machine learning models makes it difficult for users to understand how decisions are made. This lack of transparency can be a barrier for businesses that need to explain their fraud detection processes to regulators or customers.
6. Conclusion
AI is playing an increasingly critical role in the detection and prevention of fraud across industries. By leveraging machine learning and deep learning algorithms, AI-driven systems can detect fraudulent activity with greater accuracy, speed, and scalability than traditional methods. As fraud schemes continue to evolve, AI will remain a vital tool in helping organizations stay ahead of fraudsters, protect customer data, and reduce financial losses. However, businesses must address challenges related to data quality, privacy, and integration to fully harness the potential of AI in fraud detection. Ultimately, AI’s ability to adapt, learn, and analyze large datasets in real-time makes it an indispensable tool for combating fraud in the digital age.